Affective Attention-Based BILSTM Models for Personality Prediction
Keywords:
Personality prediction, MBTI, VAD analysis, Affective Attention mechanism, Ensemble, Bidirectional LSTMAbstract
Objective: This study aims to develop a novel, more accurate personality prediction model that integrates affective dimensions and reduces computational complexity.
Methods and Materials: The proposed model preprocesses textual data using a novel method that removes noise and enriches semantic features with syntactic information. GloVe word embedding is utilized for semantic representation, and Bidirectional Long Short-Term Memory (BILSTM) networks extract contextual values. Affective dimensions (valence, arousal, and dominance) from the NRC Lexicon are used to create attention layers that focus on emotional aspects of words. The ensemble of these models improves prediction accuracy. The model was evaluated using the MBTI dataset from Kaggle, comprising 8675 users' Twitter data.
Findings: The proposed model achieved an average accuracy improvement of 3.35% to 6.23% compared to state-of-the-art models like BERT while reducing execution time significantly (by 1/24 of BERT’s time). Incorporating affective dimensions enhanced predictions, particularly in personality traits resistant to change, such as Thinking-Feeling and Judging-Perceiving.
Conclusion: The integration of affective attention mechanisms and enhanced preprocessing methods significantly improves personality prediction models. The proposed approach not only boosts accuracy but also reduces computational demands, making it highly applicable in practical scenarios like customer profiling and recommendation systems. Future work could explore additional personality-related dimensions and unsupervised learning techniques.
Downloads
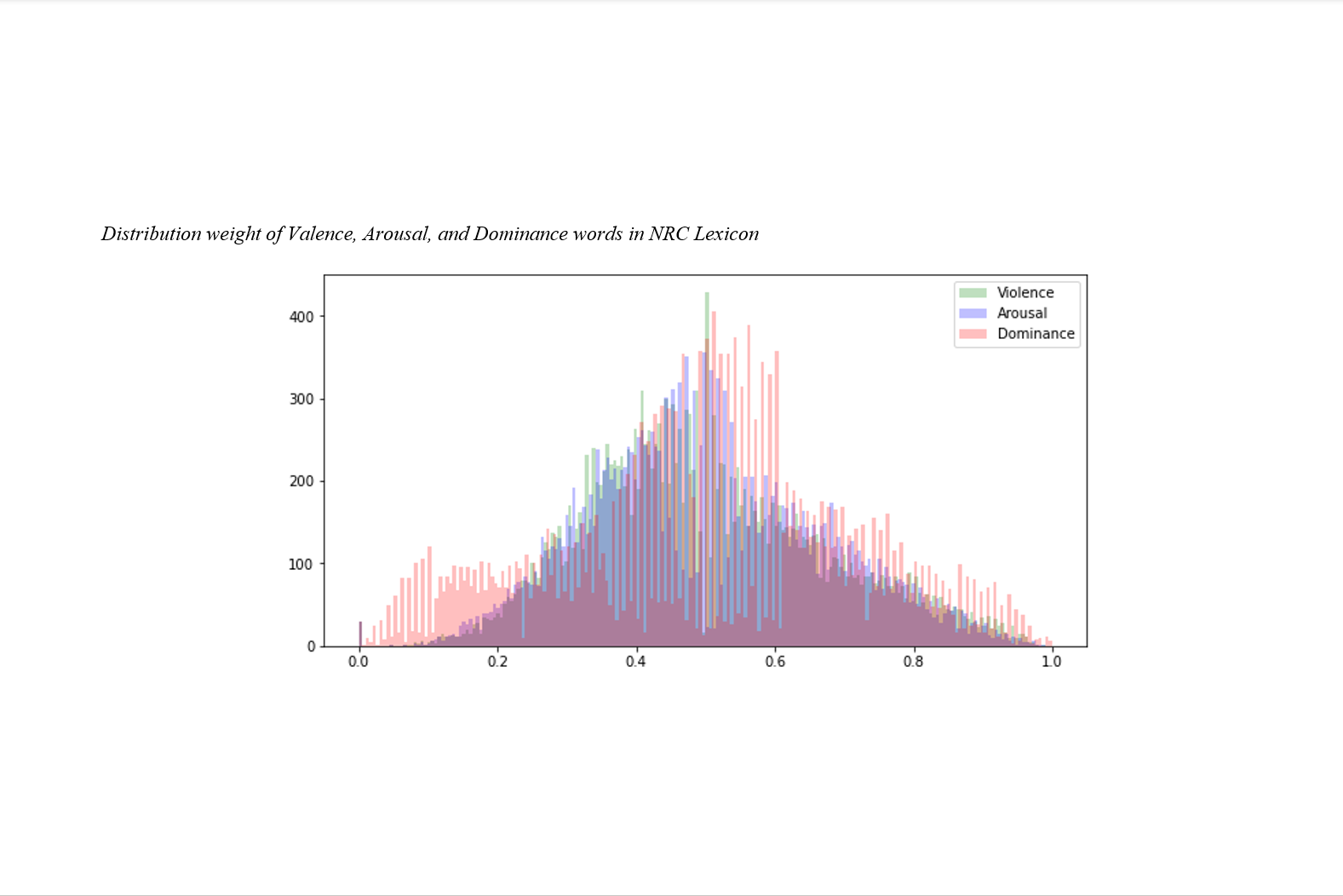
Downloads
Additional Files
Published
Submitted
Revised
Accepted
Issue
Section
License
Copyright (c) 2024 Zohreh Anisi Hemaseh (Author); Mohamad Ali Afshar Kazemi (Corresponding Author); Ehsan Mousavi Khanghah (Author)

This work is licensed under a Creative Commons Attribution-NonCommercial 4.0 International License.