Identification and Control of Credit Risk in Banks Utilizing New Supervisory Technologies with Neural Network Algorithm and Random Forest Algorithm
DOI:
https://doi.org/10.61838/kman.aitech.3.1.7Keywords:
Credit risk, bank, credit facilities, neural network algorithm, random forest algorithmAbstract
The purpose of this study is to identify and control credit risk in banks utilizing new supervisory technologies with the neural network algorithm and the random forest algorithm. This research, in terms of its nature and objective, is categorized as theoretical and applied research. Given the quantitative nature of the study and the use of data mining for customer credit scoring, this investigation is data-driven. The primary foundation of this research is the discovery of knowledge from banking databases. In this study, real customers who received credit facilities from Tejarat Bank and Saman Bank in Tehran over a one-year period, whether they returned the loans to the bank or not, were defined as the statistical population. Consequently, for sampling, all individual credit customers of the selected branches of these banks during the specified time frame were examined. Out of 500 credit customers, a simple random sampling method was employed to select those who had received loans during this period, resulting in the selection of 230 samples for this study. After collecting the previous bank customer data from the relevant database and cleaning the data, the influential variables in customer ranking were identified by reviewing previous scientific research. In the next phase, using the neural network algorithm and the random forest algorithm, and with the help of relevant software, customers were classified based on their characteristics, and their behavior was predicted. The findings indicated that the random forest algorithm was more efficient in predicting customer credit risk. Statistical test results showed that the support vector machine model had higher accuracy in predicting customer credit risk. The random forest (DT) algorithm used in this research had the highest accuracy among all models, and with feature selection, the model's accuracy increased compared to the base model, achieving the highest accuracy (81.49%) among all techniques
Downloads
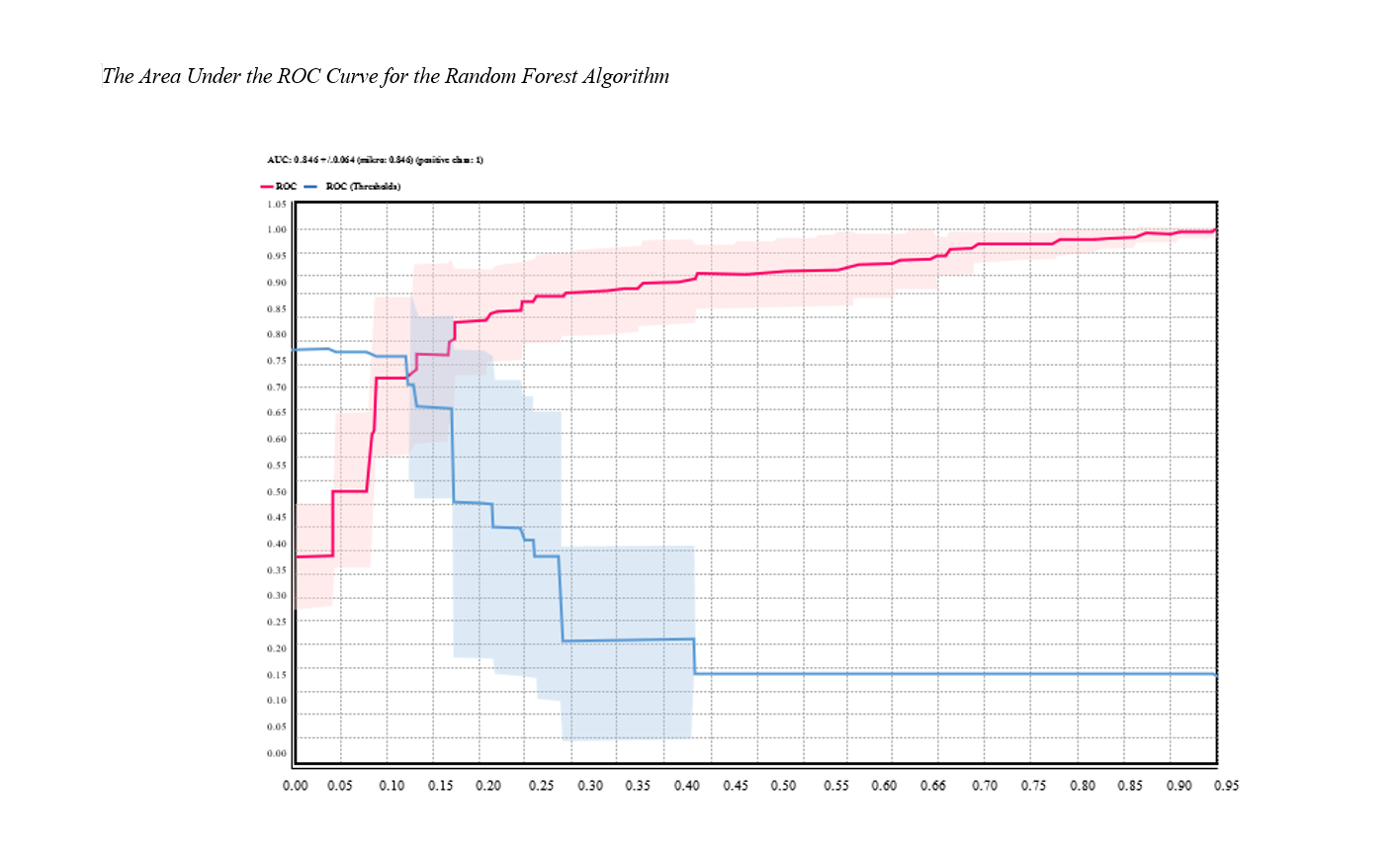