Prediction of Diabetes using Supervised Learning Approach
Keywords:
diabetes prediction, diagnosis, data mining, algorithmsAbstract
This paper provides an in-depth evaluation of various supervised machine learning models used for predicting diabetes. It discusses the strengths and limitations of several algorithms, including Decision Trees, Random Forest, Rotation Forest, Ensemble Classifier, K-Star, Simple Bayes, Logistic Regression, Functional Tree, and Perceptron Neural Network. The study utilizes a publicly available diabetes dataset from chistio.ir, which includes 520 samples, comprising 200 diabetic patients and 320 non-diabetic patients, and assesses 16 features. Results are validated on the Weka 3.6 open-source platform, using metrics such as AUC, classification accuracy (CA), F1 score, precision, and recall.
Downloads
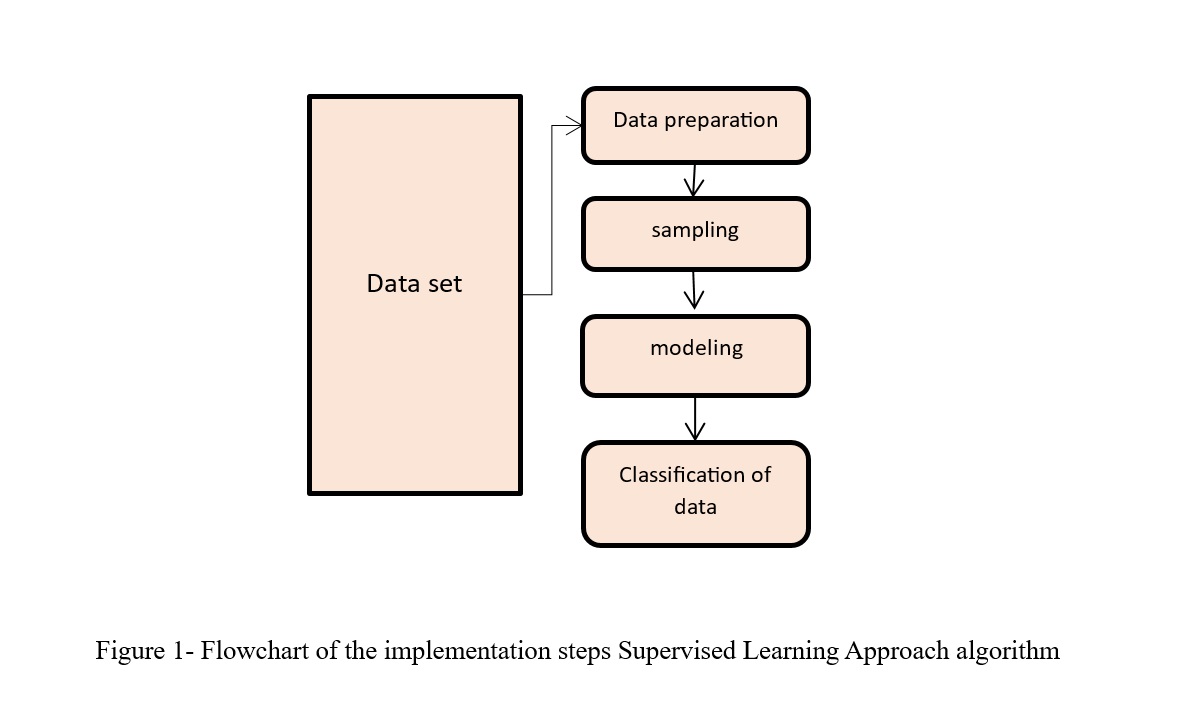
Downloads
Additional Files
Published
Issue
Section
Categories
License
Copyright (c) 2024 Nasim Khozouie (Corresponding Author); Omid Rahmani Seryasat, Sadegh Moshrefzadeh (Author)

This work is licensed under a Creative Commons Attribution-NonCommercial 4.0 International License.