Designing an Intelligent System for Vaccine Supply Chain Management Based on Blockchain Using Machine Learning Algorithms
Keywords:
Vaccine, Blockchain, Machine Learning, Supply ChainAbstract
Objective: The objective of this study is to design and evaluate an intelligent vaccine supply chain management system (VSC) based on blockchain technology, integrating machine learning algorithms and Internet of Things (IoT) devices.
Methodology: The research employs a multifaceted approach, incorporating blockchain technology to ensure data immutability and transparency, machine learning models for predictive analytics, and IoT devices for real-time monitoring. Key methodologies include the implementation of a hybrid LSTM-CNN model for credibility assessment, an SVM-based intelligent inoculation module, and sharding for improving blockchain scalability. Data for model training and evaluation were sourced from existing vaccine supply chain records and simulated scenarios to ensure robust testing of the system’s components.
Findings: The study demonstrates that integrating blockchain with machine learning significantly enhances the management of vaccine supply chains. The hybrid LSTM-CNN model effectively assesses the credibility of vaccine manufacturers, while the SVM model accurately predicts the need for vaccinations. Sharding improves the system’s scalability, allowing for efficient processing of large transaction volumes. The system also ensures that expired vaccines are promptly detected and removed from the supply chain, thereby maintaining vaccine quality and safety.
Conclusion: This research highlights the transformative potential of combining blockchain technology, IoT, and machine learning in vaccine supply chain management. The proposed system not only improves efficiency, transparency, and security but also addresses the scalability challenges inherent in global immunization efforts.
Downloads
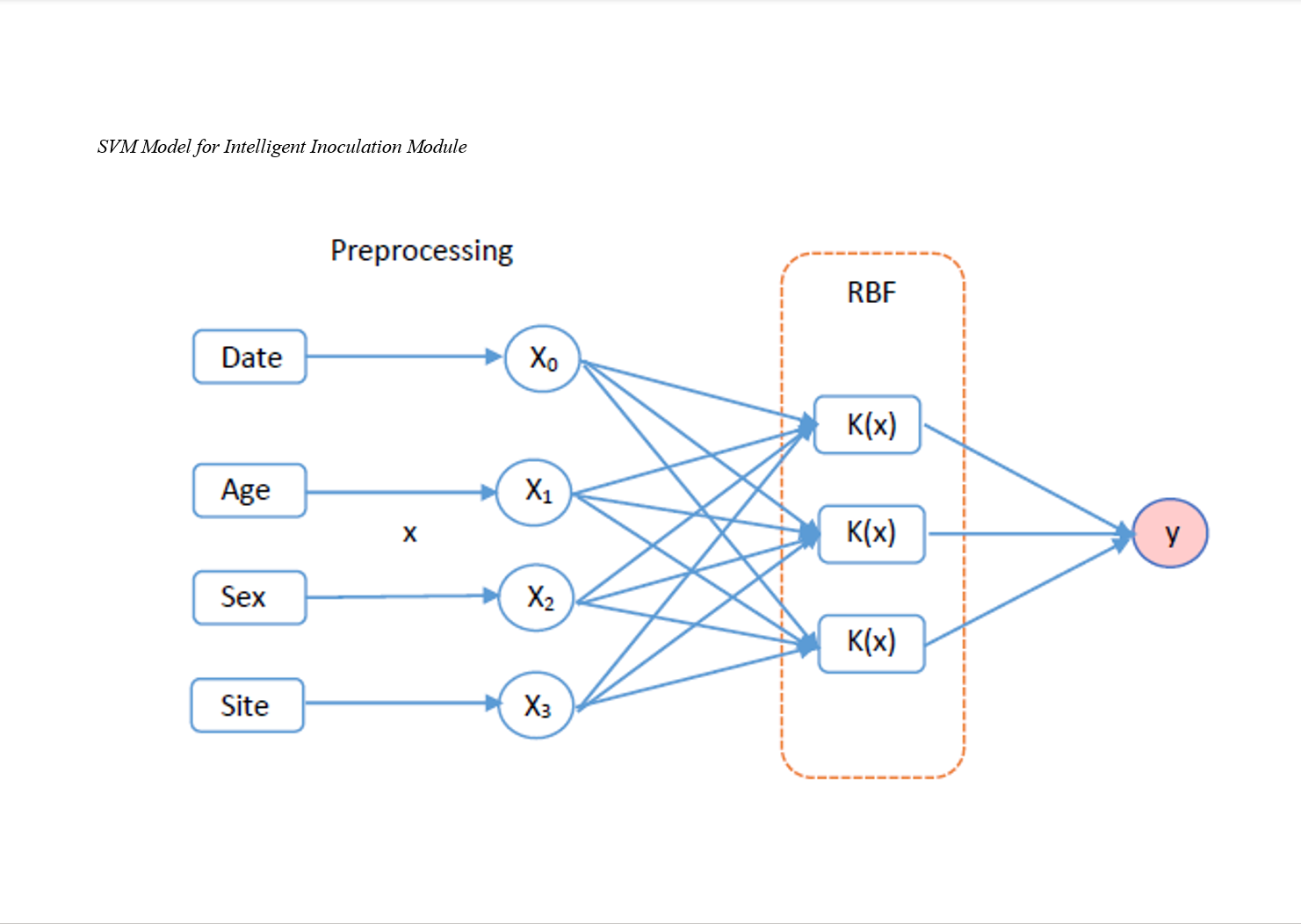
Downloads
Additional Files
Published
Submitted
Revised
Accepted
Issue
Section
License
Copyright (c) 2024 Morteza Ahmadi (Author); Shaghayegh Sahraei (Corresponding Author); Sepehr Ghazinoory (Author)

This work is licensed under a Creative Commons Attribution-NonCommercial 4.0 International License.